- HOME
- Behind the Build: Suresh Kumar B on the value of no-code machine learning
Behind the Build: Suresh Kumar B on the value of no-code machine learning
- Last Updated : October 19, 2023
- 898 Views
- 6 Min Read
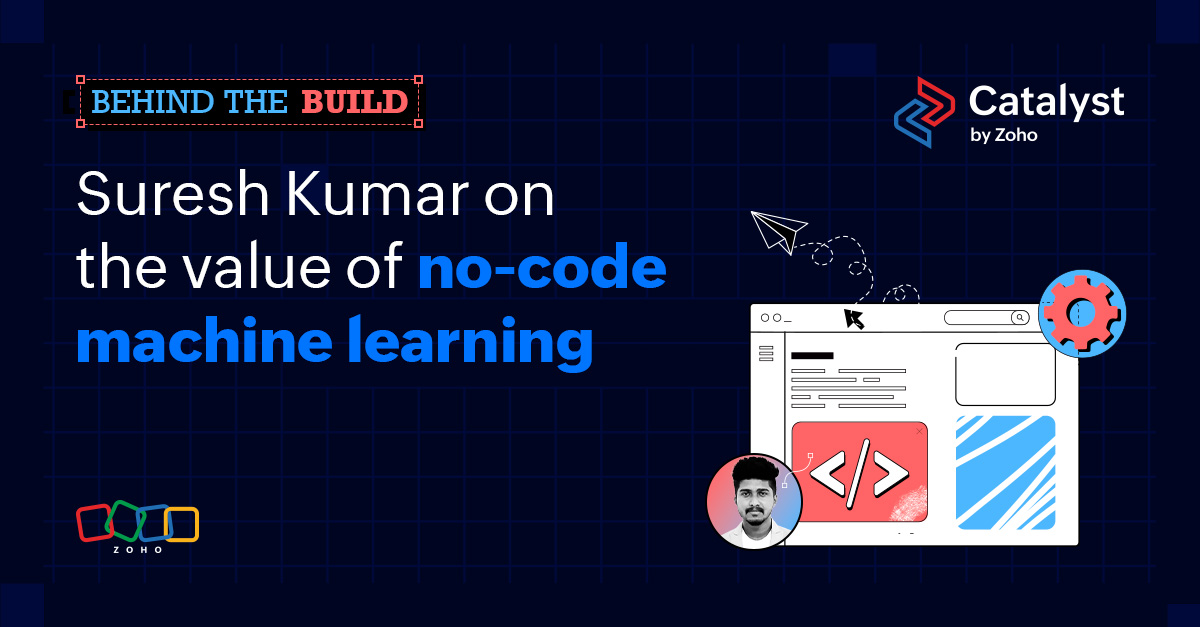
Every developer is unique, but the majority of them share a common trait: they are curious problem-solvers driven by a genuine passion for creating meticulously crafted solutions. In this no-nonsense Q&A series, we interview our in-house engineering team to gain insight into how they go about solving problems for fellow developers. The series aims to not only bring you educational content, but also foster transparency between our development team and users like you, establishing a stronger connection.
Let's meet the dev!
Featured here is Suresh Kumar B
Suresh Kumar Balasubramani is an engineering lead with a passion for crafting sophisticated software architectures for machine learning and data technologies. He played a crucial role in developing QuickML, an innovative no-code machine learning platform that simplifies machine learning for a wide range of users. His dedication to democratizing AI and data insights to make them more accessible to businesses is at the core of QuickML's mission.
Q 1: What is the inspiration behind QuickML? Who is it for?
Suresh: The primary inspiration behind QuickML is to address the gaps in the machine learning development domain in multiple areas. Usually, ML development involves working with multiple frameworks, libraries, and tools, making it complex and difficult to achieve an end-to-end ML development flow. With QuickML, we aim to make it simpler for developers to analyze, transform data, develop, run ML experiments, store output models, and manage resources. It is designed for ML developers who want a simpler, but comprehensive platform for their ML model development needs.
From our experience and market research, we found that most solutions available today are complex to use or pertain to only one area where an ML developer could not fulfill the end-to-end ML development flow with ease. Also, most of the platforms require coding and few offer no-code methodologies with fewer customization options. So, we designed an end-to-end machine learning platform that can perform all stages of machine learning operations in one place, from data preprocessing to model deployment.
Q 2: What sets QuickML apart from other low-code or no-code machine learning platforms on the market, and how do you hope developers benefit from it?
Suresh: QuickML stands out by providing a no-code approach that empowers not just ML developers, but anyone with access to business data and a desire to leverage AI and infuse AI into their business to experiment and build their own ML models. With its user-friendly drag-and-drop interface, QuickML eliminates the requirement for programming skills, making it accessible to a wider range of users. This accessibility enables seasoned and citizen developers alike to prototype swiftly and iterate on ML models, bypassing the need for extensive coding. As a result, QuickML accelerates the development process and facilitates efficient experimentation and refinement of ML solutions.
Q 3: Can you discuss the level of control and flexibility that QuickML offers to developers who prefer to customize their machine learning workflows or code?
Suresh: QuickML offers developers a remarkable level of control and flexibility through its no-code builder. Users can perform a wide range of actions and customize their machine-learning workflows by simply dragging and dropping nodes. The system's configuration editor allows users to provide minimal inputs, which are then translated into underlying ML code. This code is executed on QuickML's dedicated servers, which are equipped with the necessary resources. Whether it's filling empty values during data pre-processing or configuring hyper-parameter values for ML algorithms, QuickML serves as a comprehensive and centralized platform for all customization needs.
Q 4: How should developers assess the potential impact of adopting a no-code machine learning platform like QuickML on their team's productivity and time-to-market?
Suresh: When evaluating the potential impact of adopting a no-code machine learning platform like QuickML on their team's productivity and time-to-market, developers should consider the amount of effort and time required to build a custom ML workflow for their business. This is a critical factor, because such platforms significantly reduce the time and effort needed in complex ML and DevOps domains to set up custom ML models for production. By eliminating extensive coding and streamlining the development process, QuickML enables developers to expedite their projects and enhance overall productivity.
Q 5: Hypothetical scenario: Say you are a developer looking for a machine learning solution. What important questions would you ask yourself to evaluate if the solution is right for you and your project?
Suresh: As a developer looking for a machine learning solution, I would ask myself the following questions to evaluate if a solution like QuickML is right for my project:
- How easy is the platform for me and my team to understand and use?
- What level of control and features does the platform offer? Can it accommodate your specific requirements?
- Does the platform provide options for experimentation and comparison of different ML operations?
- How seamless is the adoption process after setting up a custom ML model? Is it easy to integrate into your existing systems or workflows?
- What options are available for post-production monitoring and feedback systems? Can you effectively track the performance and make necessary adjustments?
When adopting machine learning, important considerations include the ease of use, customization capabilities, scalability, security, and post-production support provided by the platform.
Q 6: Building software professionally is not only about shipping quickly. It’s also about optimizing for scalability and security. What’s your take on this and how does QuickML handle it?
Suresh: Ensuring scalability and security is a key focus for QuickML. We recognize that building software professionally goes beyond shipping quickly. In the case of ML-based operations, which often involve significant computational requirements, QuickML is designed to be highly scalable. We leverage multiple cloud scaling technologies, particularly for custom model training and inferences, to ensure that our platform can handle large-scale workloads effectively.
Additionally, we understand the importance of data security and privacy. Keeping customer data secure is a critical challenge, and we have addressed it through our data multi-tenancy architectures. These architectures are specifically designed to maintain the security and privacy of each customer's data. Our models are built to be highly scalable, secure, and private, providing peace of mind to our users in terms of data protection.
Q 7: There must be several exciting things you’re seeing people build with QuickML. What struck you most?
Suresh: While sales and marketing use cases are aplenty, some of our customers are using QuickML to predict employee churn. This demonstrates the platform's versatility and its potential to bring significant benefits to various business units. By infusing machine learning into HR processes, businesses can make data-driven decisions, optimize employee retention strategies, and enhance the overall efficiency of their Human Resource departments.
The ability to predict employee churn not only helps HR teams proactively address attrition but also positively impacts other operating units that rely on employees for product quality and customer service. By having a deeper understanding of employee retention patterns, companies can take appropriate measures to retain top talent, leading to improved customer satisfaction and productivity.
QuickML's no-code approach empowers line-of-business users, including HR teams to focus on more strategic tasks rather than being overwhelmed by complex data analysis. This allows them to allocate their time and resources more efficiently, ensuring the organization benefits from their expertise in managing and developing human capital. Overall, using QuickML for employee churn prediction showcases the platform's potential to leverage data insights to enhance workforce management and create a more productive and harmonious work environment.
Q 8. What do you want to build next? What are you currently working on? Looking ahead, what are the future plans and enhancements you have in mind for QuickML that will further benefit senior developers and decision-makers?
Suresh: We are currently working on including other interesting verticals on ML problem solving with our current no code approach. There are wide range of cases to cover to help businesses solve their ML requirements. Some interesting features are visual analytics of data and model-related metrics, Auto-generated ML pipelines and more granular model deployment handler and so on. We are hoping to create a simple yet powerful platform to meet growing user requirements and empower businesses with easy implementation of AI into their system.
We hope you enjoyed reading this interview where Suresh Kumar B shed light on the true potential of no-code machine learning, empowering developers, data analysts, and businesses to unlock the power of their data. With QuickML's user-friendly interface and comprehensive capabilities, it's clear that the future of machine learning is within reach for everyone.
We can't wait to share more behind-the-scenes insights with you soon. Stay tuned.